February 19, 2023
Causal graphs and the back-door criterion - A practical test on deconfounding
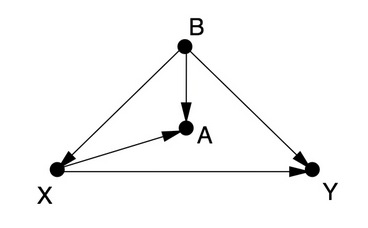
I read into causal inference recently and since I didn’t really have a use-case for it right now, I played around with some data and some causal graphs. In this article, I looked at some causal graphs from the “Book of Why” Chapter 4 by Judea Pearl and Dana Mackenzie and created simulated data based of them.
Read more